Artificial intelligence (AI) and Machine Learning (ML) are having a profound impact on the way medicine is being practiced. AI/ML algorithms and techniques fit imaging applications easily and can help with automation. Radiology is the specialty that has benefitted the most from the AI/ML revolution. Melanoma detection in Dermatology is another obvious winner.
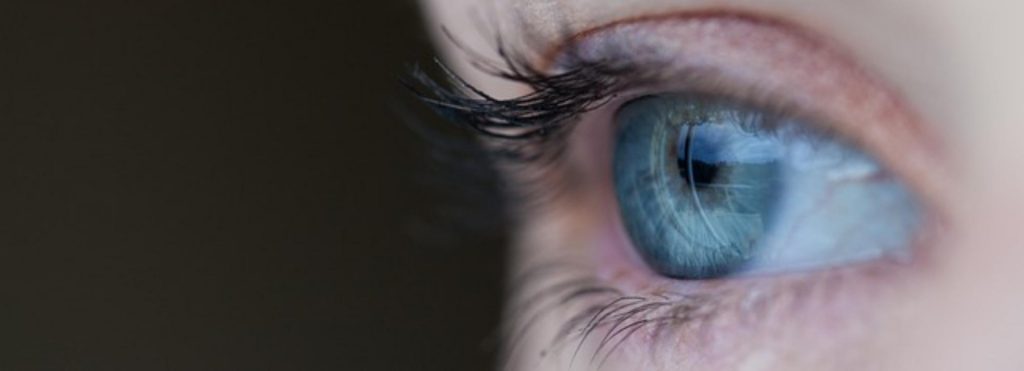
Many of the machine learning algorithms are reasonably well known. The real challenge is to get the infrastructure to crunch massive amounts of data, getting the ideal dataset for a problem, optimizing the model for performance and deploying the model for use. If you are relatively new to ML, Kaggle is a useful resource for you to start.
I will briefly introduce Kaggle for those who have not used it before. Kaggle is a platform for posting datasets that you have collected. They also provide ‘kernels’ or computational resources (typically Jupyter Notebooks) for collaborative analysis. The datasets can be made private or public under a variety of license options. Organizations post competitions and reward teams that solve them. Solutions are typically posted as predictions on a test dataset or share the kernel code
I recently noticed a good competition on Kaggle that the eHealth community may find interesting. Aravind Eye Hospital in India has posted a dataset consisting of fundoscopic images of diabetic retinopathy with varying degrees of severity. The dataset consists of thousands of images collected in rural areas by the technicians of Aravind hospital from the rural areas of India. The challenge is to develop a model that can predict the severity of diabetic retinopathy from the fundoscopic image. Further, the successful solutions will be shared with other Ophthalmologists through the 4th Asia Pacific Tele-Ophthalmology Society (APTOS) Symposium.
The competition page is available here: https://www.kaggle.com/c/aptos2019-blindness-detection
Let me know if anybody wants to team up!
- Locally hosted LLMs - July 14, 2024
- LLM-in-the Loop CQL execution - June 30, 2024
- Come, join us to make generative AI in healthcare more accessible! - June 15, 2024
Pingback: COVID vaccination tracking with blockchain - Bell Eapen
Pingback: Six things data scientists in healthcare should know - Bell Eapen
Pingback: Kedro for multimodal machine learning in healthcare - Bell Eapen